The term ‘CP3’ has surfaced in tech circles, sparking curiosity about its association with Google’s AI advancements.
In an era dominated by artificial intelligence, Google remains a key player in pushing the boundaries of what AI can do. From language models to search enhancements, Google’s initiatives have consistently reshaped the tech landscape. Amid this innovation surge, a mysterious name—CP3—has entered the conversation, leaving many wondering what it means and how it fits into Google’s broader AI strategy.
So why does CP3 matter?
As tech giants like Google continue integrating AI into everyday products and services, understanding emerging technologies like CP3 isn’t just relevant—it’s essential. Whether you’re a developer, researcher, or tech-savvy user, grasping what CP3 is could provide valuable foresight into the next wave of AI-powered tools.
In this article, we’ll unpack Who Is CP3 Google AI.
Let’s dive into the details and uncover what CP3 might signal for the future of artificial intelligence.
What Is CP3 Google AI?
CP3 stands for Cloud TP3, a cutting-edge, cloud-native AI model developed by Google AI. At its core, CP3 is a Transformer-based architecture—part of the same foundational family of models that powers well-known systems like BERT, PaLM, and GPT. However, what sets CP3 apart is its exclusive design for cloud environments, enabling scalable and optimized performance for enterprise-level natural language processing (NLP) tasks.
Google's CP3 AI isn't just a standalone marvel—it’s part of a much bigger ecosystem of advanced AI tools designed to enhance everything from productivity to creativity.
✅ Want to explore top AI tools reshaping industries? Check out our AI Tools Directory for hands-on insights.
Purpose: Why Was CP3 Developed?
The primary goal of CP3 is to enhance large-scale natural language understanding and generation. By leveraging Google’s deep learning infrastructure and cloud ecosystem, CP3 is designed to:
- Boost accuracy in NLP tasks such as entity recognition, summarization, sentiment analysis, and translation.
- Accelerate performance for real-time applications using Google’s Tensor Processing Units (TPUs).
- Offer flexibility across industries via cloud deployment, allowing for fast integration into enterprise platforms, SaaS products, and search algorithms.
Unlike general-purpose language models, CP3 is believed to be fine-tuned for enterprise-grade language operations, with enhanced control over bias, tone, and contextual accuracy—especially useful for sectors like healthcare, law, education, and customer service.
Key Features of CP3
Google’s CP3 AI model stands out not only because of its cloud-native architecture but also due to its innovative feature set. Below are the core capabilities that define its power and versatility:
Advanced Machine Learning
CP3 leverages state-of-the-art deep learning algorithms, rooted in Transformer architectures, to interpret and generate human-like language. It’s trained on diverse datasets to recognize context, sentiment, and intent, making it highly accurate in both understanding and generation tasks.
Natural Language Processing (NLP)
Built for complex language modeling, CP3 excels at a wide range of NLP tasks, including:
- Text summarization
- Entity recognition
- Sentiment analysis
- Contextual chatbot responses
- Multilingual translation
Its fine-tuned algorithms significantly reduce false positives and improve semantic comprehension, making it ideal for enterprise use.
Scalability & Cloud Integration
Being cloud-native, CP3 is optimized for deployment at scale. Whether it’s integrated into SaaS platforms, customer service systems, or search algorithms, CP3 can handle high volumes of real-time requests without sacrificing performance. It benefits from Google’s robust cloud infrastructure, including TPUs (Tensor Processing Units), to ensure lightning-fast inference speeds.
Applications of CP3
CP3 isn’t just a theoretical model—it plays a critical role in enhancing real-world AI applications across Google’s ecosystem and beyond. Here’s how it’s being used:
Search Engine Optimization (SEO)
One of the core functions of CP3 is enhancing Google Search’s language understanding capabilities. By processing the nuances of user intent and contextual meaning in queries, CP3 helps deliver:
- More accurate search results
- Better ranking of high-quality content
- Improved handling of complex or conversational queries
This leads to a richer, more relevant search experience for users.
Google’s CP3 AI initiative reflects a broader trend in AI development—especially in the sales and business automation space. Today’s top-performing teams are leveraging AI not just for insight, but for actionable results and faster deal closures.
💼 Curious how AI is revolutionizing real-world sales? Check out our curated list of the Top 17 Sales AI Tools in 2025 for Teams Who Close More Deals and see which tools are already transforming pipelines.
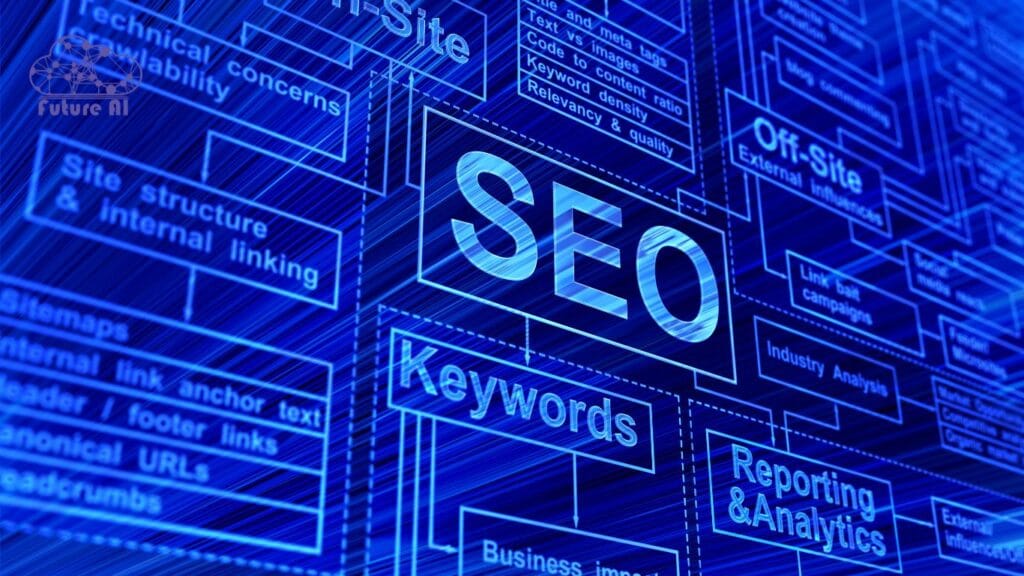
While Google’s CP3 AI focuses on next-gen artificial intelligence, its ripple effect can already be felt in the SEO landscape. From content optimization to smart keyword targeting, AI is making search performance smarter and more scalable than ever.
🚀 Want to see how AI is transforming digital strategy? Don’t miss our deep dive on the Best AI SEO Tools to Scale Agile Solutions – Automate & Optimize to discover tools that work smarter, not harder.
Voice Assistants
Google Assistant and other voice-enabled services benefit from CP3’s refined speech-to-text understanding. It enables:
- More natural interactions
- Faster response times
- Contextual memory and multiturn conversation support
This elevates the overall usability and intelligence of voice assistants, particularly in noisy environments or across different accents and dialects.
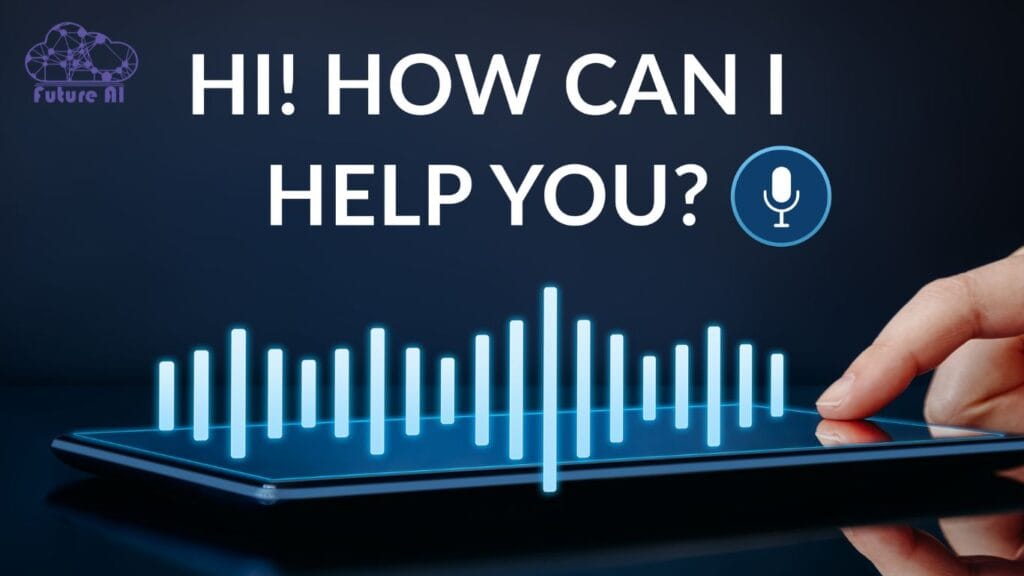
Content Moderation
With the explosion of online content, moderation is more critical than ever. CP3 aids in:
- Detecting hate speech, bullying, and misinformation
- Filtering explicit or inappropriate material
- Enforcing platform guidelines in real time
This supports safer digital environments while reducing the burden on human moderators.
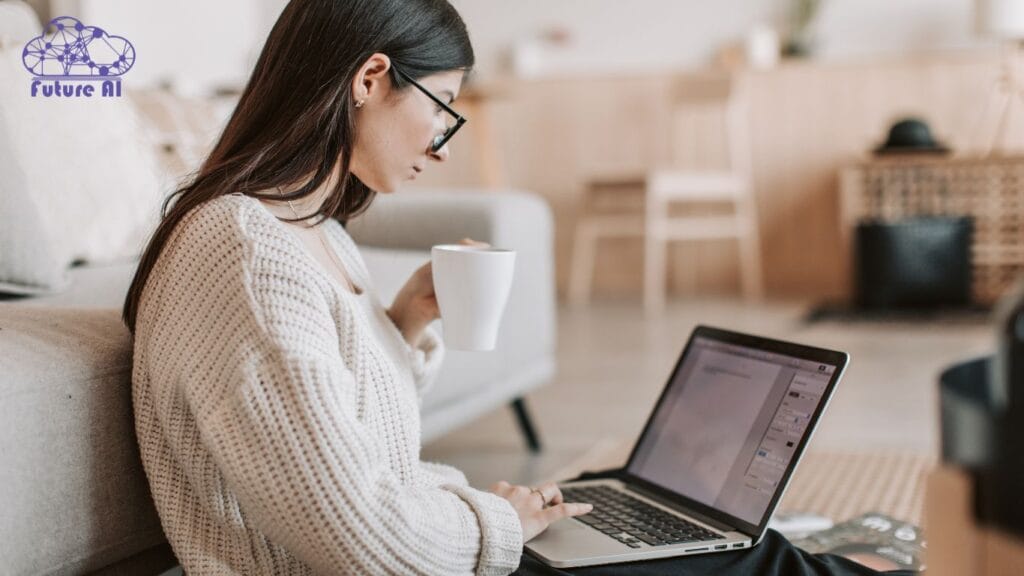
Comparison with Other AI Models
Feature/Model | CP3 (Cloud TP3) | BERT (Bidirectional Encoder Representations from Transformers) | GPT (Generative Pre-trained Transformer) |
Developer | Google AI | Google AI | OpenAI (and adapted by others like Microsoft, Anthropic) |
Primary Use Case | Cloud-scale NLP for Google Search, Voice, etc. | Search ranking, sentence-level understanding | Conversational agents, content generation |
Architecture Focus | Cloud-based, scalable processing | Bidirectional language modeling | Autoregressive (predicts next token in sequence) |
Strengths | Scalable deployment, real-time optimization | Contextual sentence understanding | Creative text generation, reasoning tasks |
Weaknesses | Less open or accessible outside Google ecosystem | Not generative, limited for longer text tasks | Can hallucinate facts, requires tuning for precision |
Applications | Google Search, Assistant, content moderation | Google Search, language understanding, SEO tools | ChatGPT, Copilot, Bard, AI writing tools |
✅ Pros and ❌ Cons of CP3, BERT, and GPT Models
Model | ✅ Pros | ❌ Cons |
CP3 | • Highly scalable via cloud infrastructure • Optimized for Google-specific applications • Real-time language processing | • Not publicly accessible • Limited documentation • Unknown adaptability for non-Google use |
BERT | • Deep contextual understanding • Excellent for sentence-level NLP tasks • Open-source and widely used in academia | • Not generative • Inefficient for large text generation • Slower inference in some tasks |
GPT | • Generates coherent and creative text • Strong few-shot and zero-shot learning • Versatile for many tasks (chat, code, etc.) | • Prone to hallucination (false facts) • Requires fine-tuning for specific tasks • Resource-intensive |
How to Implement AI Solutions Like CP3
Implementing AI solutions like CP3 (Cloud TP3) requires a solid strategy, technical know-how, and a well-planned deployment approach. Below is a step-by-step guide to help you integrate AI-driven solutions for better performance and scalability.
1. Define Clear Objectives and Use Cases
- Identify Business Needs: Determine which areas of your business could benefit most from AI, such as customer support, content moderation, voice assistants, or search optimization.
- Tailor to Specific Applications: For example, if you’re looking to enhance customer experience, consider how CP3 can improve chatbots and virtual assistants. If you want to optimize your SEO, explore its potential in improving query understanding.
2. Leverage Cloud Infrastructure
- Cloud-Based Deployment: CP3 thrives on cloud infrastructure, enabling scalability and flexibility. Start by integrating Google Cloud services to host your AI model.
- Scalability and Cost-Effectiveness: Cloud platforms provide easy scaling, meaning your AI solution can grow as your business does, without costly on-premises setups.
- Data Storage & Management: Ensure proper data storage, management, and access control when using cloud services to maintain the integrity and privacy of your data.
3. Data Preparation and Integration
- Collect Relevant Data: AI models like CP3 require large datasets for training and fine-tuning. Start by gathering structured and unstructured data (e.g., text, speech, or customer queries) that aligns with your AI application.
- Data Preprocessing: Cleanse and preprocess the data to ensure accuracy. This includes tokenizing text, handling missing values, and normalizing data for optimal model performance.
- Integrate with Existing Systems: CP3 can be integrated into existing systems through APIs or customized scripts, making the transition smoother and more cost-effective.
4. Model Training and Fine-Tuning
- Use Pre-trained Models: Leverage Google’s pre-trained CP3 models to jumpstart your project. These models come equipped with strong foundational capabilities for language processing.
- Fine-Tuning: Tailor CP3 for your specific domain or use case by fine-tuning the model. For instance, train the model to understand domain-specific terminology or respond with the right tone for customer-facing applications.
- Ongoing Evaluation: Regularly test the model with real-world data to ensure it continues to meet your performance standards.
5. Implement AI Solutions in Production
- API Integration: Integrate CP3’s functionalities directly into your products and services via REST APIs or SDKs. This allows your applications to leverage the power of AI in real-time.
- Automation: For SEO, automate content optimization, search ranking adjustments, or content moderation based on CP3’s capabilities to save time and improve precision.
- Monitoring and Feedback Loop: Set up a system to monitor the AI model’s performance and collect feedback. This will enable you to make iterative improvements over time, ensuring the solution remains effective.
6. Ethics and Privacy Considerations
- Data Privacy: Ensure that your AI models comply with data protection regulations (like GDPR). Use encrypted data storage and secure transmission channels for user data.
- Bias Mitigation: Regularly audit your AI models to identify and minimize biases in predictions. This helps in ensuring fairness and inclusivity in AI applications.
- Transparency: Maintain transparency about how the AI models work and how user data is used. This is critical for user trust and legal compliance.
7. Continuous Improvement and Optimization
- Monitor AI Performance: Implement tools to monitor the model’s performance in real time. Track metrics such as accuracy, speed, and user feedback to determine areas for improvement.
- Adapt to New Trends: Stay up-to-date with the latest advancements in AI research and upgrade your system accordingly. CP3’s evolution will likely bring new features and optimizations that could benefit your business.
- User Feedback Loop: Constantly incorporate user feedback to fine-tune the AI experience, making it more relevant and personalized for end-users.
💡 Best Practices for AI Implementation:
- Start with small, manageable projects and scale them as you gain insights.
- Collaborate with AI specialists and use online courses or documentation to deepen your understanding.
- Leverage cloud-based platforms for cost-effective and scalable deployment.
- Ensure transparency and fairness, especially if your AI models impact users directly.
By following these steps, you can successfully implement AI solutions like CP3, unlocking powerful capabilities that enhance user experience, streamline operations, and improve overall business outcomes.
Advanced Technical Details & Resources for Implementing CP3-like AI Solutions
To successfully implement AI solutions like CP3, it’s important to delve into some of the advanced technical aspects, tools, and resources that can streamline the development process. Here’s a deeper dive into these critical elements:
1. Deep Learning Frameworks for Training Models
- TensorFlow: Google’s open-source framework is ideal for developing deep learning models like CP3. It supports a wide range of neural networks and is highly optimized for cloud deployment. Use TensorFlow to create and train your Transformer-based models (similar to CP3).
- PyTorch: Another widely used deep learning framework, favored for its flexibility and ease of debugging. PyTorch can also be employed for training large-scale language models in a similar vein as CP3.
- Google Cloud AI Platform: For cloud-based deployment, use Google Cloud AI tools to scale your model. This platform helps with model training, versioning, deployment, and monitoring.
Resources:
- TensorFlow Documentation: TensorFlow
- PyTorch Documentation: PyTorch
- Google Cloud AI: Google Cloud AI Platform
2. Data Preprocessing Tools
- Natural Language Toolkit (NLTK): NLTK is a powerful Python library used for processing and analyzing text. It can handle tasks like tokenization, part-of-speech tagging, and named entity recognition (NER), which are essential when preparing data for CP3-like models.
- SpaCy: This is another advanced NLP library used for text preprocessing, including tokenization, lemmatization, and syntactic parsing. It offers high-performance features and is often used in production environments.
- Google Cloud DataPrep: Google offers a cloud-based tool to prepare and cleanse data. It automatically detects anomalies and structures datasets for optimal model training.
Resources:
- NLTK Documentation: NLTK
- SpaCy Documentation: SpaCy
- Google Cloud DataPrep: Cloud Dataprep
3. Model Fine-Tuning & Optimization
- Transfer Learning: CP3-like models can be trained using transfer learning, which leverages pre-trained models and adapts them to your specific tasks. By using Google’s pre-trained language models or OpenAI’s GPT models, you can fine-tune them to perform better for specific applications, like voice recognition or SEO optimization.
- Hyperparameter Optimization: Fine-tuning the model’s hyperparameters (e.g., learning rate, batch size, epochs) is critical for achieving optimal performance. Tools like Optuna or Ray Tune can automate this process, helping to find the best parameters for your model.
Resources:
- Transfer Learning Guide: Transfer Learning
- Optuna Documentation: Optuna
- Ray Tune: Ray Tune
4. Cloud Integration & Deployment
- Google Kubernetes Engine (GKE): For cloud deployment, use Google’s Kubernetes Engine to manage containers. CP3-like models can be deployed in a distributed, scalable manner across multiple nodes, ensuring that the system can handle high traffic and large datasets without lag.
- Serverless Deployment with Cloud Functions: Google Cloud Functions allows for serverless deployment, where you can run AI models in response to events or HTTP requests without managing the underlying infrastructure.
- TensorFlow Serving: Once your model is trained and fine-tuned, you can use TensorFlow Serving for high-performance, production-ready model deployment.
Resources:
- Google Kubernetes Engine: GKE Documentation
- Google Cloud Functions: Cloud Functions
- TensorFlow Serving: TensorFlow Serving
5. Monitoring and Model Maintenance
- Model Drift Detection: Over time, your AI model may experience “model drift,” where it performs worse as real-world data changes. Use tools like WhyLabs or Arize AI to monitor model drift and automatically trigger retraining.
- Google Cloud Monitoring: Google Cloud offers tools to monitor AI models in production, including tracking their performance, predicting issues, and identifying bottlenecks.
- A/B Testing: Implement A/B testing to compare different model versions and see how each one performs with real users. This helps refine your solution over time.
Resources:
6. Ethical AI and Bias Mitigation
- Fairness Indicators: Google’s Fairness Indicators tool helps assess bias in machine learning models. By using this, you can ensure that your CP3-like models are fair and free from unintended biases that may affect user experience or decision-making.
- Explainability Tools: Tools like LIME (Local Interpretable Model-agnostic Explanations) and SHAP (Shapley Additive Explanations) help provide insights into why your model is making specific predictions. This is essential for improving trust and transparency, especially in consumer-facing AI applications.
Resources:
- Google Fairness Indicators: Fairness Indicators
- LIME: LIME GitHub
- SHAP: SHAP
7. Training on Diverse Datasets
- Diverse Data: To make your AI models like CP3 truly powerful, you must train them on diverse datasets to understand various contexts, dialects, and user behavior. For example, if you’re using CP3 for content moderation, ensure you have a variety of text that spans different cultures and regions to avoid unintended bias.
- Synthetic Data Generation: In certain cases where real-world data is scarce, you can generate synthetic data using techniques like Data Augmentation or Generative Adversarial Networks (GANs). This allows you to enhance your model’s learning without overfitting to a specific dataset.
Resources:
- Data Augmentation with TensorFlow: Data Augmentation
- GANs for Synthetic Data: GANs for Data Generation
World Impact of CP3
The CP3 model, developed by Google, has the potential to transform various sectors by improving the efficiency and scalability of natural language processing (NLP) tasks. From business to academic research and public services, CP3 can play a significant role in optimizing workflows, enhancing user experiences, and unlocking new insights. Below are some of the key real-world applications and their impacts:
Business Applications
- Customer Service Automation: One of the most significant uses of CP3 is in customer service automation. Companies can leverage CP3’s advanced NLP capabilities to power intelligent chatbots and virtual assistants. These AI-powered systems can efficiently handle customer queries, provide product recommendations, and offer support across various communication channels such as live chat, emails, and social media. By integrating CP3, businesses can automate repetitive tasks, improve response times, and enhance customer satisfaction, all while reducing operational costs.
Examples:
- E-commerce businesses utilizing AI-driven customer service chatbots.
- Financial institutions automating routine inquiries and account management.
- Data Analysis: CP3’s ability to understand and process large volumes of unstructured data makes it an ideal tool for data-driven insights. Businesses can use CP3 to analyze customer feedback, social media content, and product reviews. By extracting actionable insights from this data, companies can make more informed decisions, predict market trends, and refine their product offerings.
Example:
- Retailers analyzing customer sentiment across social platforms to adapt their marketing strategies.
Academic Research
- Linguistic Studies: In the academic realm, CP3 can significantly enhance linguistic research by automating text analysis and providing detailed insights into language patterns. Researchers can use CP3 to study syntax, semantics, and contextual language usage in large corpora, leading to a better understanding of how language evolves and how machine learning can improve comprehension.
Example:
- Linguists utilizing CP3 for analyzing complex sentence structures in various languages.
- AI Development: As an advanced natural language processing tool, CP3 offers researchers a platform for exploring cutting-edge AI concepts. By experimenting with CP3, academic institutions can push the boundaries of AI-driven language models, develop new techniques, and contribute to the growing field of artificial intelligence.
Example:
- Universities using CP3 as a foundation for building more sophisticated AI models and conducting research in NLP and machine learning.
Public Services
- Government Services: CP3’s potential for large-scale information processing and natural language understanding can be applied to public services to improve efficiency and service delivery. Governments can utilize CP3 for tasks such as streamlining citizen inquiries, improving the accessibility of public records, and enhancing the overall communication between government bodies and the public. Additionally, CP3 can be used in emergency response systems to quickly analyze incoming data and provide timely insights to decision-makers.
Example:
- Government agencies using CP3 to automate responses to public queries related to services, policies, and regulations.
- Healthcare: CP3 can also be applied in the healthcare sector to process medical records, streamline patient communication, and assist in diagnostics. With its ability to interpret large datasets in real-time, CP3 could enhance the accessibility of medical data and improve decision-making processes for healthcare professionals.
Example:
- Medical institutions utilizing CP3 to analyze patient feedback and enhance the overall healthcare experience.
🌐 Want to see how AI is transforming real-world industries today? Explore our guide to the AI Applications in the World and discover how innovations like CP3 are part of a much larger movement.
Conclusion: CP3’s Transformative Potential
CP3 is far more than just an AI language model—its impact spans multiple industries, from business to academia and public services. By automating complex tasks, enhancing user interactions, and providing deeper insights, CP3 has the power to revolutionize how organizations operate, how research is conducted, and how public services are delivered. As the model continues to evolve, its real-world impact will only grow, offering new opportunities for innovation and improvement across a variety of sectors.
Incorporating CP3 into business operations, academic research, or public services can not only drive efficiency but also unlock new possibilities for smarter, AI-driven decision-making in the future.
FAQ Section on Who Is CP3 Google AI?
What does CP3 stand for in Google AI?
CP3 stands for “Cloud TP3,” a cloud-based Transformer-based pure-language processing model developed by Google AI. It is designed to enhance natural language understanding and process large-scale, real-time data efficiently.
How does CP3 differ from other AI models?
CP3 differs from models like BERT and GPT by focusing on cloud-based, scalable language processing. While BERT emphasizes bidirectional understanding of text and GPT is a general-purpose language model, CP3 is optimized for use within the Google ecosystem and designed for specific tasks, such as enhancing Google Search and automating content moderation.
What are the practical applications of CP3?
CP3 is applied across a variety of sectors. It is used to improve Google’s search algorithms, enhance voice recognition systems in Google Assistant, support content moderation efforts to filter harmful or inappropriate content, and can even assist in business data analysis and customer service automation.
Is CP3 available for public use?
As of now, CP3 is primarily used within Google’s ecosystem and is not available for public use. It powers various Google services and applications, but there are no plans for a general public API or independent access at this time.